Swimming in schools saves energy
Researchers at ETH Zurich have clarified the previously unresolved question of whether fish save energy by swimming together in schools. They achieved this by simulating the complex physics on the supercomputer ‘Piz Daint’ and combining detailed flow simulations with a reinforcement learning algorithm for the first time.
The schooling behaviour of fish fascinates engineers as much as biologists. Fish in schools swim in a flow environment full of mechanical energy generated by the movements of their fellow swimmers. Researchers from ETH Zurich’s Computational Science & Engineering Lab (CSElab) have now answered the longstanding question of whether fish gain an energetic advantage by swimming in schooling formations – and the answer is “yes.” Researchers also gained detailed knowledge about this process, which may have implications for energy-efficient swimming or flying swarms of drones.
Precise simulation of fluid dynamics
For their study, the scientists developed a highly detailed simulation of the complex interplay between swimming fish and their flow environment. Fish schooling was previously only tackled with very simplified models that did not account accurately for the fluid dynamics of the fish swimming. However, the supercomputer ‘Piz Daint’ at the Swiss National Supercomputing Centre (CSCS) has now enabled for the first time these state-of-the-art computationally intensive simulations without simplifications.
The scientists also combined for the first time the realistic flow simulations with reinforcement learning, a potent machine learning algorithm. This kind of learning algorithm has been used in games such as ‘Go’, enabling the computer to outperform humans. Deploying reinforcement learning on complex physical systems, however, has never been done before, as the algorithm requires thousands of iterations.
The algorithm is reminiscent of Pavlov’s dog, the scientists from CSElab say: The agents learn an optimal strategy for achieving a goal by receiving a reward. Here, it was used to train the fish for optimal swimming behaviour and to let them decide independently how to most efficiently react to the unsteady flow fields of their fellow swimmers. “We created the mathematical conditions and gave the fish the single goal of swimming as efficiently as possible,” says Guido Novati, a PhD candidate at CSElab and the developer of the simulations’ software. Surprisingly, the fish opted to swim in each other’s wake in order to save energy even when given the option of swimming independently.
Learning from the visualisation
In their simulation, the researchers observed the swimming behaviour of up to three fish, both in 2D and 3D, in various configurations. They remarked that no flow simulation has ever included more than one swimmer in three dimensions. They analysed every detail of each individual flow vortex to understand the behaviour of the fish. “Intuitively, you assume that fish will avoid turbulent areas and swim in calmer water. But instead they learned to swim directly into the vortices,” says Siddhartha Verma, a postdoc at CSElab. Verma and Novati conducted the study, recently published online in the Proceedings of the National Academy of Sciences (PNAS), under the leadership of ETH professor Petros Koumoutsakos.
The researchers determined that the fish swam most energetically when they swam not one after the other, as previously suggested, but at an offset from the swimming direction of the leader. At such locations they harnessed the vortices generated by the leader by intercepting them with their head, splitting the vortex into fragments that they then guided down their bodies. The progress of these fragmented vortices supplies the fish with thrust without robbing the leader of energy.
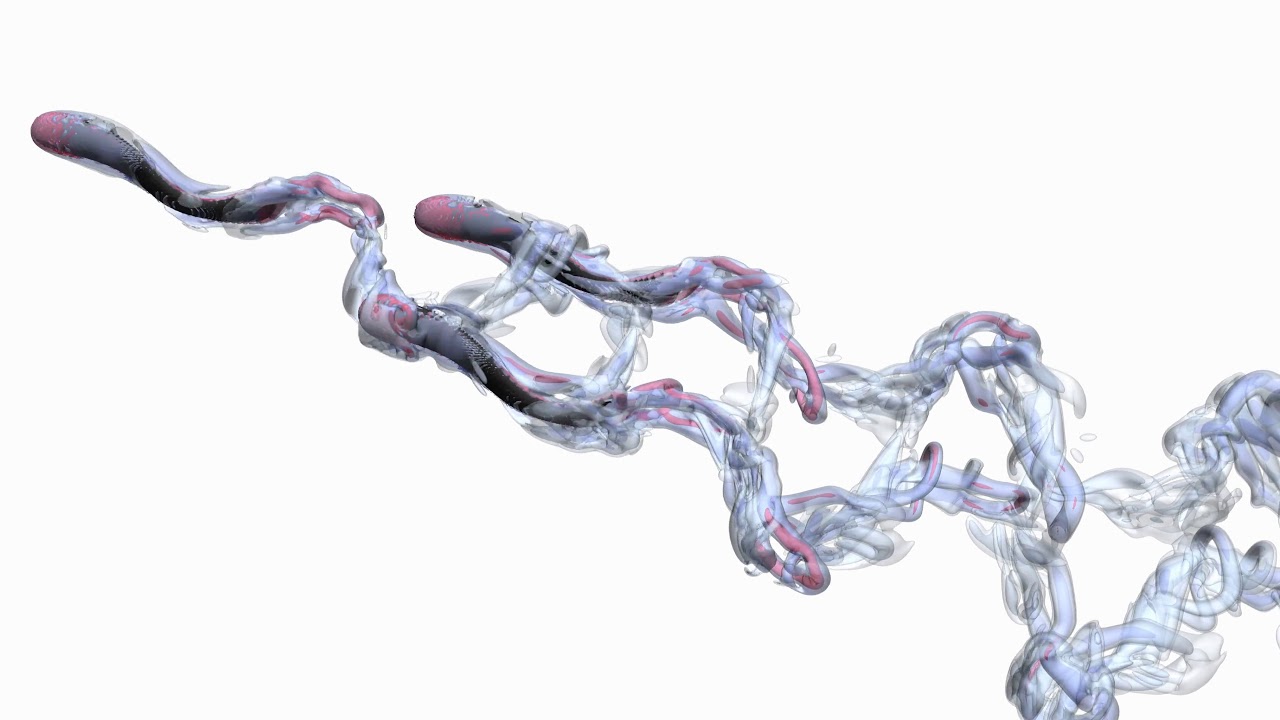
Autonomous robots
“This let us demonstrate that fish which suitably position themselves in a school can draw on energy from the prevailing fluid dynamics,” says Verma. He emphasises that in their simulations they have not examined every aspect involved in the efficient swimming behaviour of fish, but it is clear that the developed algorithms and physics learned can be transferred into autonomously swimming or flying robots.
An autonomous robot swimmer or flyer could handle unexpected flow situations – for example, when flying between buildings in high winds to deliver goods, or during search and rescue operations in stormy conditions. “The possibility of letting aeroplanes with similar destinations fly in formation along certain routes to save fuel is also being considered. The algorithms developed in our work could also be put to use here,” says Novati. The researchers are enthusiastic about the possibilities opened up by combining accurate and complex flow simulations with reinforcement learning, and they hope that in future other researchers will judiciously incorporate machine learning into their simulations.
Reference
Verma S, Novati G, Koumoutsakos P: Efficient collective swimming by harnessing vortices through deep reinforcement learning, PNAS published ahead of print May 21, 2018. external pagehttps://doi.org/10.1073/pnas.1800923115call_made
This article by Simone Ulmer, editor science and technology, has been published first on the web site of external pageCSCScall_made.